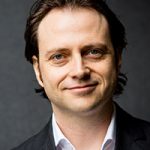
Founder of CX-AI.com and CEO of Success Drivers
// Pioneering Causal AI for Insights since 2001 //
Author, Speaker, Father of two, a huge Metallica fan.
Author: Frank Buckler, Ph.D.
Published on: September 20, 2021 * 7 min read
Do you know how to increase the fiscal impact? Expecting a great product or service without excellent customer service is like expecting your beautiful garden flowers to flourish without giving attention to them. You can increase your fiscal impact by adopting the following two methods:
- You need to be consistent in your service delivery
- You need to encourage your customers to complain
You know that NPS measures the customers’ loyalty and how likely they will refer your products and services to others. It also helps to identify the promoters and detractors of your business. If NPS is so important, you should know how to measure it.

Get your FREE hardcopy of the “CX Insights Manifesto”
FREE for all client-side Insights professionals.
We ship your hardcopy to USA, CA, UK, GER, FR, IT, and ESP.
In the earlier blog, we discussed the simulator where you can put in the fiscal impact of one person if you improve the NPS by one point. You can see the improvement in the customer value when you improve the NPS point.
You cannot simply look at the correlation between NPS and the customer value or revenue per customer (you can have any measurement of customer value). For instance, let’s take the NPS ratings of last year of a particular set of customers and check:
- Did the ratings evolve positively?
- Did the customers with lower ratings buy less? Etc.
When you do this exercise, you will often see something like the diagram shown below.
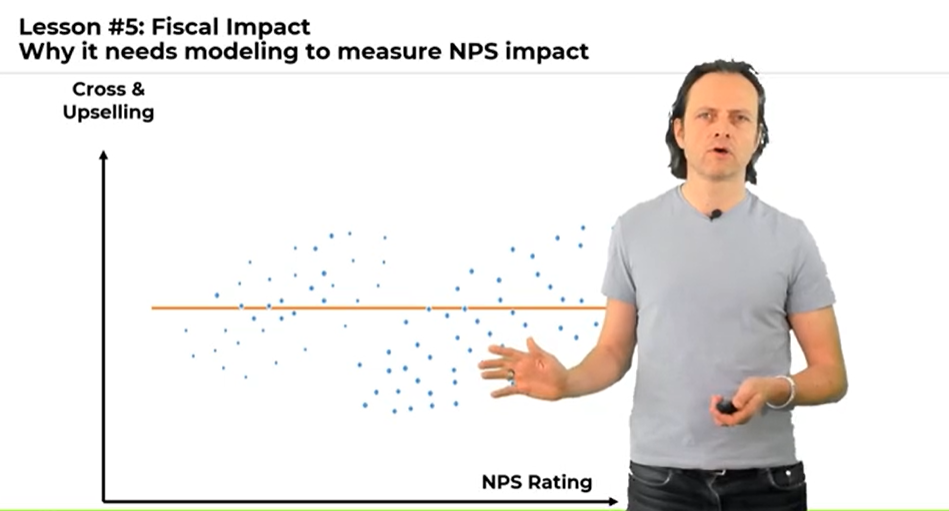
You would see no correlation between NPS Rating and the impact. It’s against all theory, and you’d be thinking why the graph is like this. It’s because it is a spurious correlation and needs modeling.
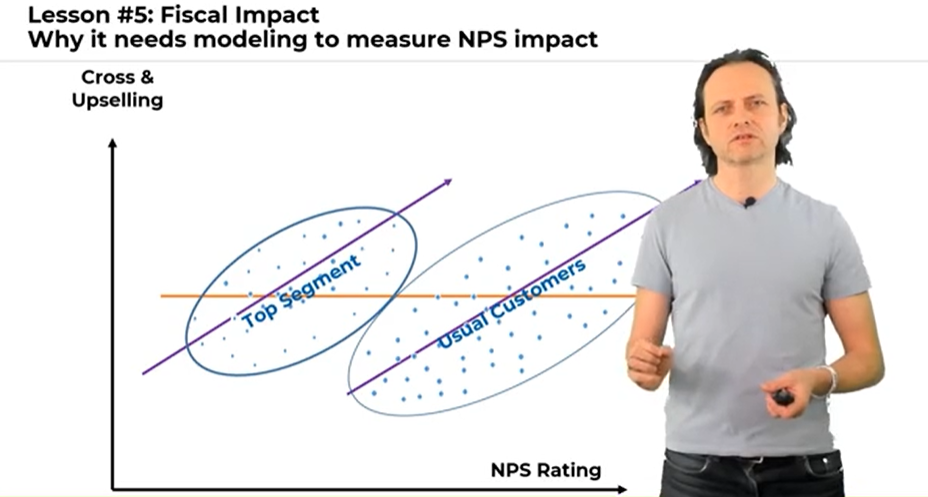
In the example above, we can see that there are two different segments:
- Top segment
- Usual Customers
You will observe that the NPS significantly impacts outcomes if you use modeling to control the segments. The Usual Customers is the segment that does not have high expectations. On the other hand, the Top Segment is the one that expects high, and because of their expectations, they, on default, give low ratings. But when they are satisfied, they are likely to buy more because they have the means for that. So, they are the customers who have the bias to rate less, but if they are satisfied, they become pretty valuable. That’s how you can use modeling to control the customer segments.
The diagram below clears the understanding between the two customer segments.

Are you aware of the importance of the fiscal impact? The fiscal impact is essential and super powerful because:
- It helps you judge the ROI ( Return on Investment) of your actions.
- It helps you compare the ROI of customer experience with new customer acquisition.
There’s an old saying that:
“Keeping your existing customers is five times more effective than gaining new customers.”
It may be a myth because it has not been proven in scientific studies. But still, it is a very fruitful exercise to have customer acquisition and experience as you need to understand the ROI.
You need to measure or assume a fiscal value of one NPS point – It’s a starting point to understand the ROI of your actions. It’s very useful to even start with an assumption. You can take your past experience or get experience from other experts and put it into the simulator (that’s an assumption). Afterwards, you can do the exercise of modeling.
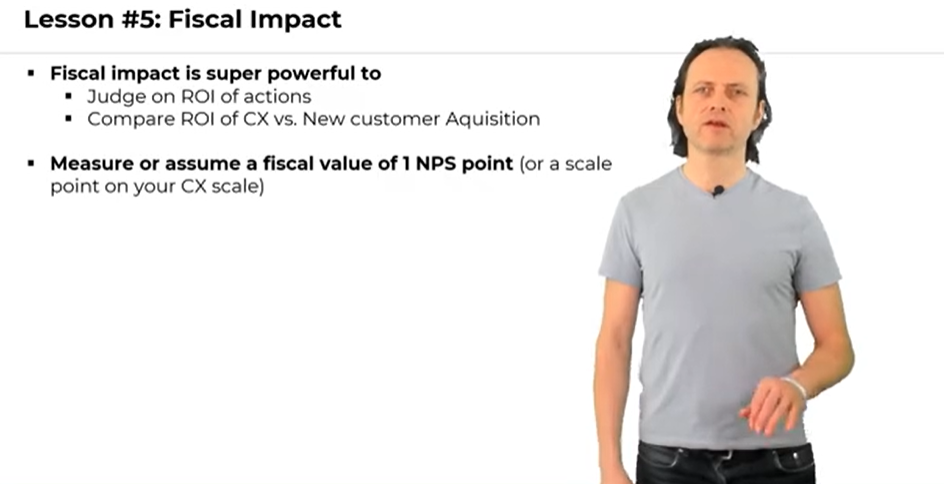
It takes a one-off modeling study to measure the fiscal impact.
- First, you need to take a past customer experience survey. Take the data from the last term and amend it for every single customer to check:
- How does the customer evolve?
- Does he churn?
- Does he buy more?
- Does he buy an additional product? What’s the margin of it?
- What’s the typical time the customer stays on this product? Etc.
2. You need to calculate the value of the churn or the customer value. You can also use past NPS ratings along with customer master data and context information to predict how many products have been purchased and if the customer has churned. You can multiply your NPS rating with the value of your action to come up with an impact on the bottom line.
3. Your past survey should ideally include different customer profile information. The more the data, the better you will have the picture of your customer. For instance, if you know where your customer lives, it will be the indicator of the potential confounder. Further, we’ll use modeling to predict the outcomes. We will use NPS and try to use all information it knows about the customer. If NPS is the signal information just really needed to predict the outcome, it can be assumed to have a true impact. So, modeling gives us an unstandardized impact value of the NPS rating onto outcomes.
4. This value needs to go into the calculation that considers:
- Product Lifetime
- Margin
- Customer Value
You need to transfer and recalculate the NPS rating towards the NPS score.
This way, the impact value can give you great insights related to the impact of your actions.


Keep Yourself Updated
On the Latest Indepth Thought-Leadership Articles From Frank Buckler
So far, we discussed that we can use modeling to measure the fiscal impact, which is important because it judges the ROI of your actions and compares the ROI of customer experience with new customer acquisition. Further, we discussed, to measure the fiscal impact, we need to do the following:
- Take a past CX survey.
- Use NPS rating and other customer data to predict the value of the churn.
- Modeling will give you an impact value of NPS.
- You need to feed this value into the calculation.
Lastly, you need to find the P&L (profit and loss) impact of our NPS changes as it will guide your investment in the right direction.
"CX Analytics Masters" Course
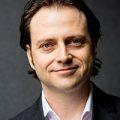
P.S. Would you like to get the complete & interactive FREE CX Measurement Guidance for your business in 2021?
Simply subscribe on the free “CX ANALYTICS MASTERS” course below and enjoy the above-mentioned training guidance in its Class # 1.





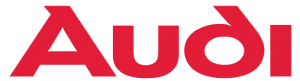
“Solves key challenges in CX analytics”
Big Love to All Our Readers Around the World
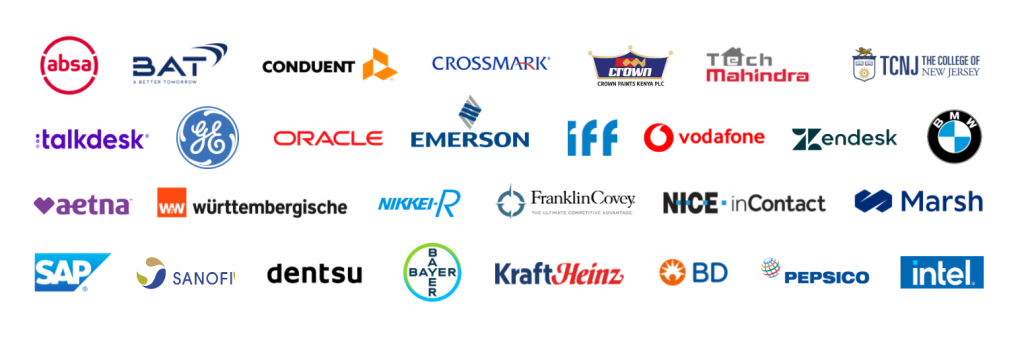
IMPRINT
Our Group: www.Success-Drivers.com
Privacy Policy
Copyright © 2021. All rights reserved.