Representativeness:
How AI can lead us out of the mess
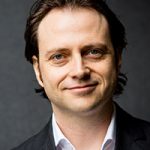
Founder of CX-AI.com and CEO of Success Drivers
// Pioneering Causal AI for Insights since 2001 //
Author, Speaker, Father of two, a huge Metallica fan.
Author: Frank Buckler, Ph.D.
Published on: November 9, 2023 * 5 min read
If you ask journalists what makes a representative survey, you often hear: it needs at least 1,000 respondents. This makes experienced market researchers smile. They know that representativeness has absolutely nothing to do with the sample size. There is no simple answer to the question of how to obtain representative studies. Not yet.
How do you know that a sample is representative? Simply check whether the demographics look like the statistics from the residents’ registration office. That sounds simple. Good luck with it!
You need a large number of respondents to obtain stable results. A representative sample, on the other hand, is needed to obtain truthful results. Stable and true are two independent characteristics. While stability is easy to establish, representativeness often gives market researchers gray hair.
Let’s assume that 100 people are surveyed. In line with official statistics, 20 percent of the sample are young people, and 20 percent are high earners. But now it may be that (for whatever reason) the 20 young people are also high earners, which would not be representative at all. Admittedly, this is an extreme example. It is only intended to illustrate: Quotas based on demographic characteristics are a blunt sword when it comes to ensuring representativeness.
But it gets even trickier. In many cases, it makes no sense at all to focus on demographics. If, for example, you want to measure a political opinion or the propensity to buy an electric car, then it is crucial that the sample is representative of the types of values prevalent in the population. This usually correlates only moderately with pure demographics.
“Ok, then I’ll use the value types” is what comes to mind. Sure, you can query value types in the screener and try to weight the evaluation according to a hopefully known distribution.
But there are two catches: firstly, I need to know what influences my desired metric for political opinion or the propensity to buy an electric car, for example. Only then can I manage this type of representativeness in advance. Secondly, I need to know the distribution of these hopefully known, moderating influences in the population.
In short, representativeness is almost impossible to control for practical market research. It’s a bit like flying blind.
The influences on representativeness are different in every study and are usually little known. Their distribution in the population is also unclear. And as a further point, I cannot ensure that the multidimensional distribution (= cross-distribution of different dimensions) is correct.

Get your FREE hardcopy of the “CX Insights Manifesto”
FREE for all client-side Insights professionals.
We ship your hardcopy to USA, CA, UK, GER, FR, IT, and ESP.
What now?
You bet… how about AI? Here is a four-step plan that was created entirely without AI
- Measure the representativeness drivers
You should think in advance about which aspects could influence the key metric. The emphasis is on “could”, because we often don’t know exactly. The idea is to cast a wide net and then, in step two, look at this wide net to see what actually has a significant influence. These aspects are then added to the questionnaire.
- Modeling with Causal AI
Now we calculate a flexible driver model (ideally with Causal AI) and find out whether part of the variance in the target variable(s) of the study can be predicted by the representativeness drivers. If so, it is important to manage representativeness in these variables. We are interested here in the effect size of the representativeness drivers, not the effect size. In the case of non-linearities or interactions, there is a big difference here.
- Ground truth
The search for other representative studies can help to find the right population for the relevant representativeness drivers. Alternatively, you can also try to derive or triangulate plausible values from secondary sources. In case of doubt, data from expert opinions or prompting a LLM is better than nothing.
- Shortcut: Simulate with AI
With the help of the driver model from 2. and the ground truth information, the target value distorted by the non-representative sample can be corrected. All you have to do is change the measured values of the representativeness drivers in the data set to correspond to the ground truth information. The driver model uses these correlated corrected values to calculate a target value that is closer to the truth.
- Simulate virtual respondents with AI
The shortcut corrects the distribution of a variable, but it does not solve the problem of multivariable maldistribution (= incorrect cross-distribution of variables). This can only be solved with the help of a representative base sample. By a representative base sample, I mean a sample that is collected using random routes and face-to-face interviews and stratification by area. The variables that the base sample has in common with the current study can now be used in the model of 2. The comparison with the current sample can measure the bias effect, and thus, this bias can be eliminated. There is not enough space here to describe this procedure in detail.
How do we do this in concrete terms?
Jenni Romaniuk, Associate Director of the Ehrenberg-Bass Institute, said in her keynote speech at the planung&analyse Insights conference in Frankfurt: “Don’t set quotas for demographics but for past brand usage”. The first rethink must, therefore, take place when recognizing the drivers of representativeness.
Our experience from hundreds of causal driver analyses also shows this: Demographics often show no or only moderate influence. Composing a sample with people who have very different levels of experience with a brand can completely distort the results.
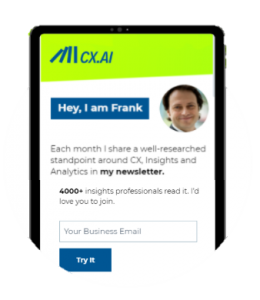
Keep Yourself Updated
On the Latest Indepth Thought-Leadership Articles From Frank Buckler
My take-away
Companies and market researchers need results they can trust. That’s why they make sure that the panel quality is right and that the data is collected in a “reasonably” representative way. We have just explained how difficult this is. A certain resignation has already crept into the industry.
This is not necessary. At Microsoft, for example, we were able to accompany a process in which the global customer satisfaction surveys are viewed in such a way that the key figures are comparable between different waves and fluctuate less. Previously, key figures for segments and markets were only reported from a sample of N=100. Today, N=50 is sufficient. We were able to show in studies that the recalibrated values are closer to the truth than the raw data with a smaller sample size.
We therefore recommend systematically monitoring the representativeness drivers of the studies with flexible causal AI driver models – i.e. not with conventional multivariate statistics. An automated analysis process would be desirable. Not every company has the capacity that Microsoft does. Perhaps an innovative start-up will soon be found to tackle the issue.
Who knows? Your thoughts?
"CX Standpoint" Newsletter
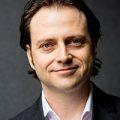
Each month I share a well-researched standpoint around CX, Insights and Analytics in my newsletter.
+4000 insights professionals read this bi-weekly for a reason.
I’d love you to join.





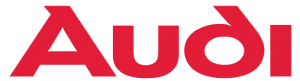
“It’s short, sweet, and practical.”
Big Love to All Our Readers Around the World
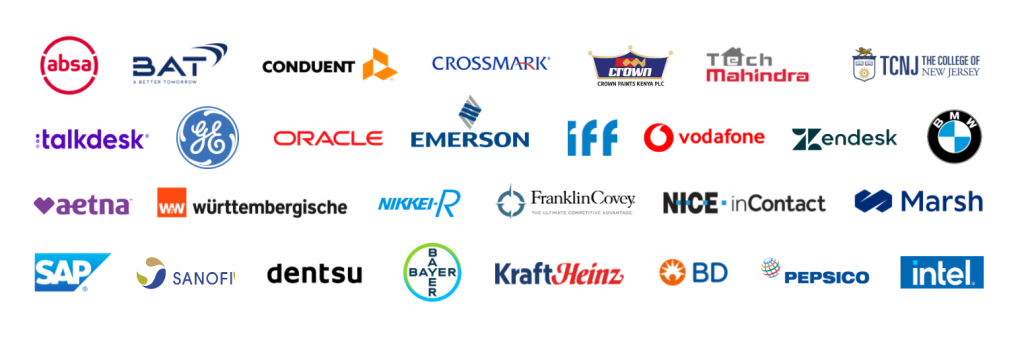