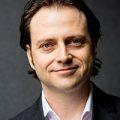
Each month I share a well-researched standpoint around CX, Insights and Analytics in my newsletter.
+4000 insights professionals read this bi-weekly for a reason.
I’d love you to join.





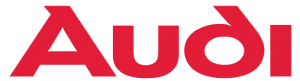
“It’s short, sweet, and practical.”
CX.AI -Experience the new generation of CX Insights
Correlation is not causation — but what is causal?
Founder of CX-AI.com and CEO of Success Drivers
// Pioneering Causal AI for Insights since 2001 //
Author, Speaker, Father of two, a huge Metallica fan.
Author: Frank Buckler, Ph.D.
Published on: 15.04.2021 * 9 min read
Correlation, causation, statistics — all this sounds boring, complicated, and not practical. I’ll prove in this article that NONE OF THIS IS TRUE.
Since the beginning of humanity, we have roamed through savannahs and ancient forests and gained causal insights day in day out.
One tried to light a fire with sandstone — it didn’t work. One used a sharp stone to open the Mammut — worked. One tried these red berries — died within one hour.
Correlation works excellent in simple environments. It works great if you have only a handful of possible causes, AND the effect is following shortly after.
Fast forward, one million years: Day in day out, we are roaming through leadership zoom meetings and business dashboards.
“David did this, next year sales dropped. Let’s fire him.”. “NPS increased, great job our strategy is working”.
Is it really that easy?
We still use our stone-age methods. We use them to hunt for causal insights and to justify the next best actions. Action that costs millions or billions in budget.
FREE for all client-side Insights professionals.
We ship your hardcopy to USA, CA, UK, GER, FR, IT, and ESP.
If you invest today in customer service training, you will not see results right away. It may even get worse for a while. Later dozens of other things will impact the overall outcome — new competitors, new staff, new products, new customers, new virus mutation, or even a new president.
You cannot see -just by looking at it- that an insight is wrong or right. Even if you put the insight into action and try it out, you will not witness if it works or not.
Dozens or hundreds of other factors influence outcomes. Even worse, activities take weeks, months, or years to culminate into effects.
I believe people know this. But they don’t have a tool to cope with it. This is why everyone goes back to Neanderthal modes — like a fly, hitting the window over and over again, just because it knows no better way.
It was a sunny September day in 1998. I was sitting in my final oral exam of my master diploma with Professor Trommsdorff — THE leading Marketing scientist in Germany at that time.
He was asking me, “What are the prerequisites for causality?” I answered what I had learned from his textbook:
Even during this exam, I knew that this definition is useless for real life.
Here is why…
Point #1 — Correlation: most NPS ratings do NOT correlate with resulting customer value. We can still prove a significant causal effect. Below you will find a great example of why it is. Correlation is NOT a prerequisite of causality. This is only true in controllable laboratory experiments.
Point #2 Theory: How can you unearth new causal insights if you always need to have a supporting theory? This is just useless for business applications. Actually, it’s also holding back progress for academia too.
One underlying reason for this useless definition is that academia has different goals than businesses. Academia aims to find the ultimate truth. As such, it wants to set more rigid criteria (spoiler: this helps for testing but not exploring causality).
For businesses, the ultimate truth is not relevant. Instead, what you want is to choose actions that are more likely to be successful and less likely costly.
Because today “Causality” is associated with “ultimate truth”. Academia is avoiding this word like the devil in the holy water — from statistics all the way through marketing science.
Because science is largely neglecting causality, it is not correctly taught in universities and business schools.
This then is why businesses around the world are still in a Neanderthal mode of decision-making.
Question: What are the most crucial business questions that need research? Is it like how large a segment or market is (descriptive facts), or is it which action will lead most effectively to business outcomes?
Exactly, this is the №1 misconception in customer insights. Everyone expects that “insights” are unknown facts that we need to discover.
In truth, these crucial insights are mostly not facts but the relationship BETWEEN the facts that a business is looking for. It’s the hunt for cause-effect insights.
But how can we unearth such insights?
Here is a practical causality understanding that enables the exploration of causal insights from data. At its core, it relies on the work of Clive Granger. In 2000 he was awarded the Nobel Prize for his work.
In 2013 we took a look at brand tracking data of the US mobile carrier market. T-Mobile was interested to find out why its new strategy was working. The question was: is it the elimination of contract terms, the flat fee plan or the iphone on top that attract customers?
Causal machine learning found that NONE of the many well-correlating factors had been the primary reason. It was the Robin-Hood-like positioning as the revolutionary brand “kicking AT&Ts bud for screwing customers”.
A “driver” is causing an “outcome” directly if it is mutually “predictive”. It means that when looking at all available drivers and context data, this particular driver data improves the ability of a predictive model to predict the outcome. So did the new positioning perception for T-Mobile.
If every driver correlates with outcomes, the model may need just one of all drivers to predict the outcome. This one driver is -proven by Granger- most likely the direct cause.
95% of new product launches in grocery do not survive the first year — although brands have professional market research departments.
We let causal machine learning run wild on a dataset with all US product launches, its initial perception, ingredients, pricing, brand, repurchase rate, and then the effect to survival and sales success.
Our client was desperate as nothing was correlating and classical statistical regression had no explanatory power.
It turned out that reality violates rigid assumptions that conventional statistical models require. Machine Learning suddenly could very well predict launch success with 80% accuracy. It even could explain it causally. What it takes to launch success is to bring ALL success factors in good shape. You cannot compromise on any of them.
The product needs to be in many stores (1), the pricing must be acceptable (2), the initial perception must be intriguing (3) and the product must be good to cause repurchases (4). Only if all comes together, the product will fly.
A driver is causal if it is predictive. Now Machine Learning enables us to build much more flexible predictive models. We don’t need to assume anymore that those factors add up (like in regression).
We can have Machine Learning find out how exactly the cause enfolds its effect. No matter if additive, multiplier type, nonlinear saturation or threshold effect, Machine Learning will find it in data.
If the predictive model is flexible e.g. it can capture previously unknown nonlinearities, it improves predictability. That’s what AI and Machine Learning can do today.
Coming back to the T-Mobile example. None of the new features had been found to be the direct cause of success. Does this mean they had been useless?
Not at all. The new features like “no contract binding” were reasoning the Robin-Hood-perception. The feature perception proves to be predictive for positioning perception. This is called an indirect causal effect.
A driver can cause the outcome by indirectly influencing the direct cause of the outcome. That’s why you need a “network modeling” approach.
The whole philosophy of regression and key driver analysis is a simple input-output logic — and it leads to bad, biased, misleading results.
…we should use them as a last resort only.
Often we see that NPS ratings do not correlate with increased customer value. The picture below shows the data points of customers. On the horizontal axis is the NPS rating and on the Y-axis the change in cross and upselling afterwards.
Overall, both data do not correlate. That’s what we actually see in most datasets. NPS has a hard time correlating with Cross & Upselling as well as Churn. But not because it doesn’t work.
Often there are high-value segments that tend to be more critical when rating. When the rating improves, the cross & upselling increases even more as these are high-income segments.
Within each segment, the NPS rating correlates, overall it does not correlate.
If your causal model would not have the segment information and if it would not have as well other information that correlates with the segment, THEN ….
…your model is only true with the assumption that no significant third factors (so called “confounders”) influence cause and effect at the same time.
Granger called this in his work “the closed world” assumption.
There is a last causal assumption to discuss:
Let’s take NPS rating data again. You could be tempted to take it and correlate or model it against the customers’ revenue.
Customer revenue is an aggregate of the last year’s purchases but NPS is just the loyalty of now. Such analysis would assume that the present can cause the past.
Of course you need to make sure that by any means the cause is likely happening before the effect.
Often, we even do not have time-series data. Then you need to judge in the causal direction using other methods, such as PC-algorithms used in Bayesian networks, or additive noise modeling methods, or as a last resort, an assumption based on prior knowledge.
When I speak about causality in talks, I typically hear the objection: “yes, but it’s impossible to be sure that those two assumptions have met.”
Fair point. But what’s the alternative?
Guesswork?
BS storytelling?
Back to Neanderthals spurious correlations?
This is so hard to accept: while insights about facts are obvious, insights about (cause-effect) relationships can NOT ultimately be “proven”. You need to infer them from data.
When doing so the only thing you can do is to make LESS mistakes.
Latest Causal Machine Learning methods enable us to:
Leave Neanderthal times to the past and take the latest tools and become plumper of insights 😊
The good news is…
You can NOT make a mistake by just starting to improve.
The benchmark is not to arrive at the ultimate truth. That’s an impossible and impractical goal. The benchmark is to get insights that are more likely to drive results.
Causation is an endlessly important concept that everyone seems to avoid — simply because it’s not understood.
You can drive change by educating your peers, colleagues and supervisors. The first step is to share this article. 😉
“There is nothing more deceptive than an obvious fact”
Sherlock Holmes
Literature:
Buckler, F./Hennig-Thurau, T. (2008): Identifying Hidden Structures in Marketing’s Structural Models Through Universal Structure Modeling: An Explorative Neural Network Complement to LISREL and PLS, in: Marketing Journal of Research and Management, Vol. 4, S. 47–66.
Granger, C. W. J. (1969). “Investigating Causal Relations by Econometric Models and Cross-spectral Methods”. Econometrica. 37 (3): 424–438. doi:10.2307/1912791. JSTOR 1912791.
Each month I share a well-researched standpoint around CX, Insights and Analytics in my newsletter.
+4000 insights professionals read this bi-weekly for a reason.
I’d love you to join.
“It’s short, sweet, and practical.”