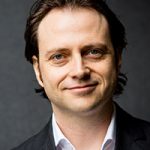
Founder of CX-AI.com and CEO of Success Drivers
// Pioneering Causal AI for Insights since 2001 //
Author, Speaker, Father of two, a huge Metallica fan.
Author: Frank Buckler, Ph.D.
Published on: April 05, 2022 * 5 min read
Claudia worked on this piece of analytics for the last 6 months. It was nerve-wracking. She was working for a large global provider of syndicated market research that had assembled a mind-blowing dataset: Data about all new product launches in CPG in the US. Details product perception and presales purchase intent, sales data, distribution data, everything.
Claudia’s task was to build a mechanism that predicts -based on presales shopper assessments- whether or not a product will sell and survive.
Nothing worked. Purchase intent correlated with success ZERO. Regression delivered R2 close to ZERO. Then, some destiny let the company reach out to us at Success Drivers.
We ran our holistic causal machine learning approach and achieved great explanation power but something was still odd. Typically, the method gives great transparency about causal relationships, nonlinearities, and two-way interactions of any kind.
We paused. The look at the skewed distribution of success (very few are very successful) brought the Eureka moment. Such a distribution evolved only if you multiply four or more independent success drivers. Its rare that all 4 hit the mark at a time, so it becomes rare that a product survives the first year after lunch.
Suddenly all this made sense. You will not survive with bad packaging or messaging, hardly with a missing brand. You will not survive if the pricing is not appropriate. You will not survive if the product is not that great, so people don’t want to rebuy it. You will not survive if retailers do not put the product on their shelves.
There are so many MUST Dos, it is likely that you miss just one of them. If you do, you fail.
Methodically speaking, this is a 4-way interaction – the success can be computed by multiplying success drivers variables. Causal machine learning can learn this out of data.
Later, I realized this learning applies to some degree to all business success drivers. Drivers seemed to work indeed independently on an incremental/micro level, but not on a macro level. This is why we can get easily fooled.

Get your FREE hardcopy of the “CX Insights Manifesto”
FREE for all client-side Insights professionals.
We ship your hardcopy to USA, CA, UK, GER, FR, IT, and ESP.
The other year we were starting to promote a new insights product and launched a new website for it. Thru multiple channels, we were planning to drive traffic to it. But the website was not converting into demo requests.
We hired conversion experts, UX specialists, and multiple star copywriters. We optimized and ran A/B testing. It got better and better. We thought. Actually, the performance stayed very bad.
Then I talked with Pedro – a Conversion rate expert – and he gave me the Eureka moment. “Your website is not the problem,” he said. It seemed that the audience does not resonate with the offering.
Clear if the audience you attract is not exactly those people who may need your product, the website cant convert. If your product is not solving an obvious pain point of the audience it will have a hard time ever converting.
The greatest website of all times will not sell, if your product doesn’t solve an urgent need.
It’s like riding a dead horse. Lipstick on a pick. Success Drivers do not compensate each other, they multiply. Any multiplication with zero, stays zero.
Senior leadership use to ask “how important is x or y”. The answer is always “it depends”. Even worse, the truth about the importance constantly changes.
If you fix the pricing of your product, it may still not fly, simply because you still have to manage that retailers put it again back on the shelves.
If you fix your biggest bottleneck the next bottleneck pops up soon.
Like a water hose with multiple holes. If you fix one hole, the others start leaking even more. Until you fixed them all.
Let’s take Customer Experience Management. If your processes do not work, your apps crash, your telephone routes people nowhere, when the basics are misaligned, everything else is not important.
Success Drivers multiply. Any multiplication with zero stays zero.
It’s fair to assume that every business is operating a chain with crucial elements. The weakest link defines the total chain’s performance.
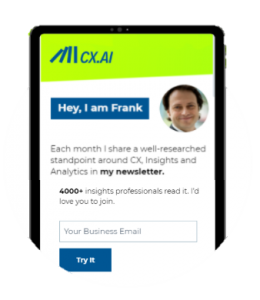
Keep Yourself Updated
On the Latest Indepth Thought-Leadership Articles From Frank Buckler
When you next time tries to measure the importance of success drivers, think twice.
Most methods out there are assuming that success drivers are independently important. No matter if you run a regression, econometric modeling, Bayesian nets or you name it.
Maybe they allow for build-in assumptions on interactions/multiplications – but you need to know upfront which one. This is an unrealistic ask that 99% of businesses cant specify upfront.
Using cause machine learning (at CX-AI.com we use NEUSREL neusrel.com) instead gives you the full flexibility to discover “what is” instead of “what should be”.
What are your experiences in this?
Write me!
Frank
"CX Standpoint" Newsletter
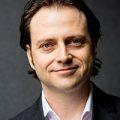
Each month I share a well-researched standpoint around CX, Insights and Analytics in my newsletter.
+4000 insights professionals read this bi-weekly for a reason.
I’d love you to join.





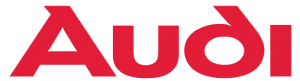
“It’s short, sweet, and practical.”
Big Love to All Our Readers Around the World
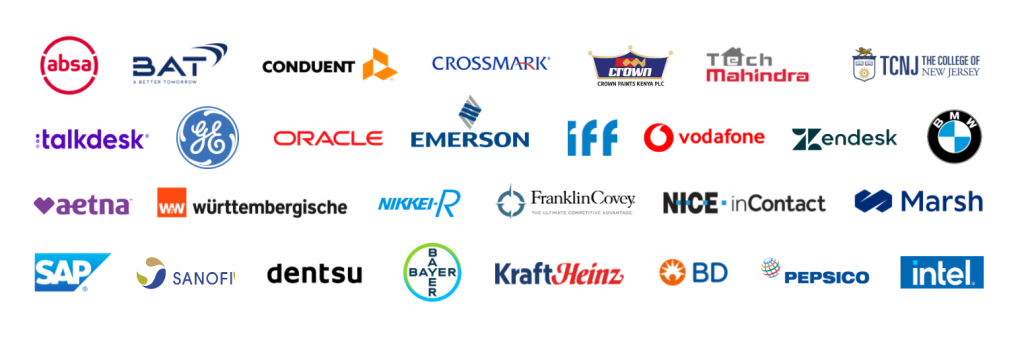