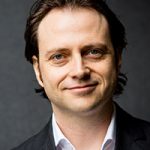
Founder of CX-AI.com and CEO of Success Drivers
// Pioneering Causal AI for Insights since 2001 //
Author, Speaker, Father of two, a huge Metallica fan.
Author: Frank Buckler, Ph.D.
Published on: October 19, 2021 * 14 min read
A customer experience score helps you get the average satisfaction score of the customer. For instance, in an automated survey, customers rate their specific experiences like a service call or a product purchase on the scale of “very satisfied” or “not satisfied at all.” The CX scores can fluctuate due to several reasons. Let’s discuss those reasons first.

Get your FREE hardcopy of the “CX Insights Manifesto”
FREE for all client-side Insights professionals.
We ship your hardcopy to USA, CA, UK, GER, FR, IT, and ESP.
Following are the reasons due to which the CX scores fluctuate.
- Market dynamics – The CX scores fluctuate as there is a movement in your customer experience either through the competition or what is happening in your organization. There is a natural dynamic in the market, so the CX score does not stay stable.
- Sample Size – The noise that occurs due to the fluctuating CX scores can be huge for several reasons. The first reason is the sample size. Many people think that the CX score can become stable if the sample size is doubled. However, it is not the case. To get double stability, you need four times more i-e., 4X sample size. This way, you will reduce your variance to half.
The sample size is NOT the merit and solution to everything. Instead, we need to look at:
- What can we do with the sample size?
- How can we extract more information from the sample size?
- Ratio Score – One of the noises and variations comes from the way we compute the score, especially NPS that is a ratio score and not the mean. It takes ratios of promoters, subtracts the ratios of detractors, and ratios the same way as the TOP2 boxes are very fragile towards low sample sizes. So, they have a high variance. Why is it?
Imagine you have a sample size of a hundred, and the typical share of promoters is just 5%. So, you will expect five out of a hundred to fall into the promoters. If two are missing for some reason, you suddenly have a 40% less score in the promoter piece.
If the same happens with the detractor, many more people are in the neutral zone. So, there will be a variation due to this small change. The scores will be largely different. As the ratio scores are always more fluctuating, so the NPS is an unstable score.
- Weighting – Another factor that makes the CX score even worse is weighting. Many companies take their fluent or high-value customers and overweight them by the factor of 10 (let’s say). But if you have a low sample size, it is another reason for the fluctuation.
In short, weighting multiplies the ratio effect and amplifies the whole measurement problem of NPS.
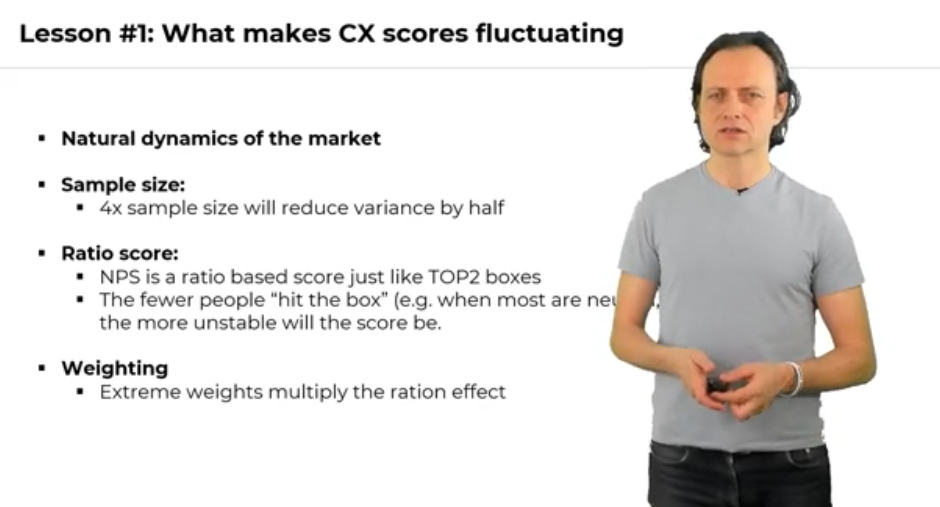
Let’s discuss some simple tactics to mitigate the effect. They are as:
- Use Fuzzy Logic – Instead of computing the NPS, the standard way is to use fuzzy logic. It is not complicated. If you understand everything, it’s simple.
In the below chart, you can see the different scores of NPS.

The blue points indicate how you treated the different NPS scores. If someone is six or lower (detractor), you treat them as minus a hundred percent NPS because across all the customers, you set an average and get the NPS score.
On the other hand, you treat promoters as hundred percent NPS because if everyone is a promoter, the average is hundred percent. In the seven lines, you treat them as zero. That’s basically how the NPS score calculation treats responses. It’s a binary thing i-e., bad, and good. You can use fuzzy logic and say, for instance, age is not the same as seven. It is positive neutral.
In the above chart, seven is a negative neutral, and nine is positive but not a hundred percent, maybe 70 or so. You may ask how to know which value to take. You can assume some facts and can see if, on average, the same NPS score emerges across many different times or splits. You can try it out, and you will find those measurements that pretty much, on average, give the same NPS. But for this specific moment, it gives a different NPS because it acknowledges that seven is NOT eight, and your customers did not mean eight though you treat it the same way.
So, fuzzy logic makes more sense of what your customers are saying, but you still get an NPS score.
- Boost Sample – You can boost the sample. For instance, for the extreme weights or the high-value customers, you can try to boost the sample and reach more often out to them or reach a larger sample. So, you get more feedback from high-value customers. For instance, if something is double in value, you can reach out to double per person.
- Moving Average – You can compute the moving average with the past value. Why? You may say you can not average out the new value with the old one. It would be a wrong value because neither the old nor the new value are the true values. Both are highly affected for low sample size by noise. You can filter out this noise by simply meaning them out.
- Weighted Average – It’s a simple technique, but if you want to use some other tactics, you can mean the noise with some benchmarks. If the general trend is upwards, there is a chance that the specific trend is also upwards.
- Simulation Exercise – Anything you do should be simulated because you might say:
“I can not compute the average from the old with the new value.”
How to know that it will be better and not be worse. You only know if you do the simulation. Remember, your score is NOT the truth. It is highly fluctuating through biases, i-e., through a sample bias, through weighting, through measurement, etc.

Let’s discuss how to calibrate your KPIs using modeling. Typically it is advised to use machine learning as a modeling technique because it is more flexible, has higher predictive power, and assumes fewer assumptions. The idea is that you take your score and try to predict it. For instance, you can predict the NPS score of fluent customers in Germany. Then, you can have another split like retails in Switzerland. So, whatever splits you have, you can have the score.
Predictors – You try to predict which score you can expect if you know its predictors. Some of the predictors are as:
- Score last term – The first predictor is the score of the last term. There is a strong autocorrelation of scores. If there is a high loyalty in the USA, it will be the case for the next period. There might be a change, but this is the biggest information needed to predict that the score is the score of the last term.
- Score before the last term – You can also include the score before the last term and can find out when the last time the outlier occurred.
- Score change of other segments – You can put the change of the score in other segments. The scores in other segments are widely different. Some are high, some are low, but it does not matter for this score. But if there is a typical change in other segments, it might be predictive for the score (predicted score) as shown below. Typically, the customer serves the same product to all the segments or all regions.

- Score change of other regions – If there is a new product or a new service initiative, it impacts too many segments or too many regions, and typically they are correlated. The score changes for not only the segments but also for the regions.
- Sample Size – Low sample size means a high variation or high change from past to future.
- Mean not score – You should try using this score instead of using the score of the last term. It is because you don’t want to report the mean of the NPS. After all, it is hard to interpret for decision-makers. But actually, it is a more stable score, and we can use machine learning to translate it into the future score. That’s the beauty of machine learning, as we don’t need to know how all the predictors interrelate. Often, there are strong interactions among the scores when conventional statistics do not work at all. It typically gives half of the predictive power, so we need to leverage machine learning here.
- TWIN splits – Average, not all others but use most correlating TWIN splits. Instead of looking at the change of all other segments, you can find out which segments typically correlate with the existing segment. You need to use the correlation matrix and find out which split correlates with the other split. So, you find TWINS, and these TWINS are to be more predictive to each other.
- Other indicators – There might be other indicators about the splits like sales number, churn, etc. These are the real-world numbers that are the indicators of what is happening in the real world for your customers. So, whatever numbers you have, they can go into the modeling, and machine learning will find out if they are useful or not.
CAUTION:
Not use other items of the same survey.

When you do an NPS survey, you can have items like service product, pricing, etc. You can take the average score of the segment for quality service (let’s say) as a predictor for the final score. It is a good predictor, but it will still fool you. Why?
Imagine you have a low sample size and have fifty respondents in one split. The main reason for the fluctuation is sampling, how they fall into the NPS pockets, and weighted. The sample bias, along with the weighting bias, applies to the items like quality, product service, etc. So, if you have some strange people in the sample who screw up your score, they will also be the reason who screw up these items. You see, we cannot predict the truth, and that’s what we want to know.
So, the above caution will help predict the scores, but it will not help predict the ultimate truth because the score is again biased. Therefore, you need simulation for the calibration modeling to find out the truth.

SPEED-TRAINING: Reinvent Your CX Analytics and Win C-Suite by Storm
Crystal Clear CX-Insights with 4X Impact of Actions
60-minute Condensed Wisdom from The World's #1 CX Analytics Course
*Book your slot now, last few seats left*
Whether simple or ML-based, all calibration needs proof through sampling, and given below is the method you need to use.
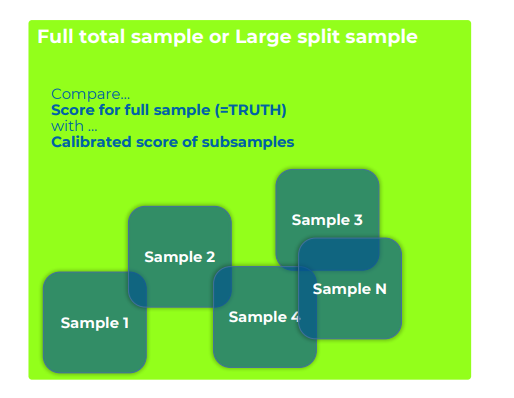
You take a split that is large enough. If you don’t have large splits, you can take the whole sample. An example of a large split is retail customers in the USA. You can do sampling with this split. For instance, if you have a 5000 or 10,000 sample score, you are pretty close to the truth. Out of this thousand or more, you can subsample now like 100, 10, 20, 30, 50. You can take out some samples and calibrate them.
You try to make the sample score better and compare it with the overall score of the sample because you know it is pretty close to the truth.
Consider an example below.

What you see on the left is the score change with and without calibration. Here the NPS score for a hundred or fewer samples variates. Typically it can vary between -20 and +20 for a low sample size. So, the calibration gets much more stable.
On the right, you can see the simulation result. The blue line indicates the sample size, and the orange line is the standard error or the deviation from the truth. There are jumps in the simulation chart if the sample size is below a hundred. Even if it is a hundred, it has a typical fluctuation of five. So, it depends on your weighting and score scheme. It can be quite high, and you wouldn’t expect that it’s so high.
The orange line indicates the status quo, and the blue line is the calibrator. So, you learned if you have 20 or 30 respondents, the blue line can be as stable as 150. Now, you can report splits as low as 25 samples.
You see that the calibrated line is always better than the actual line because the measurement is just a measurement. It’s an estimation and not the truth. So, we just want to calibrate it towards the truth, and the sampling exercise tells us whether or not we are on a good track.
So far, we discussed that scores built from the limited sample size strongly fluctuate around the truth. Part of the problem is the way we calculate the scores like ratio or weighting scores. Further, some easy fixes can make the score more stable. For instance, fuzzy logic can help mean out the actual versus last score.
But the most efficient and the most precise way is to use machine learning. It is the most powerful way to bring every score closer to the truth.
"CX Analytics Masters" Course
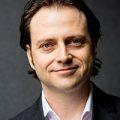
P.S. Would you like to get the complete & interactive FREE CX Measurement Guidance for your business in 2021?
Simply subscribe on the free “CX ANALYTICS MASTERS” course below and enjoy the above-mentioned training guidance in its Class # 1.





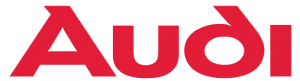
“Solves key challenges in CX analytics”
Big Love to All Our Readers Around the World
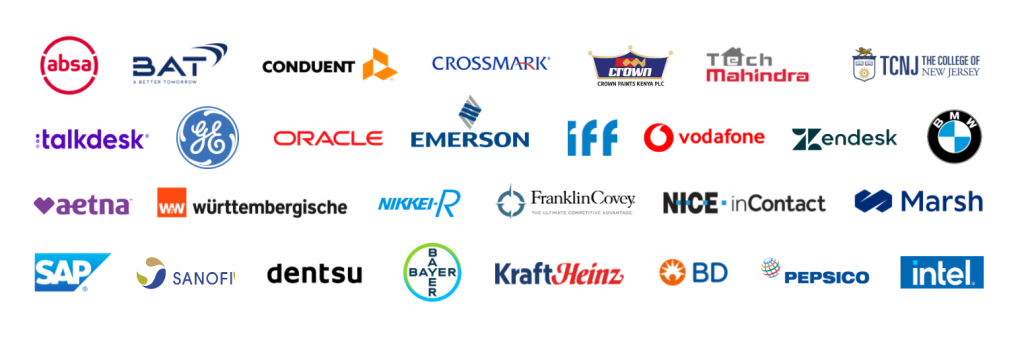
IMPRINT
Our Group: www.Success-Drivers.com
Privacy Policy
Copyright © 2021. All rights reserved.