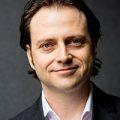
P.S. Would you like to get the complete & interactive FREE CX Measurement Guidance for your business in 2021?
Simply subscribe on the free “CX ANALYTICS MASTERS” course below and enjoy the above-mentioned training guidance in its Class # 1.





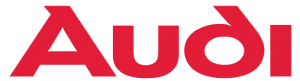
“Solves key challenges in CX analytics”