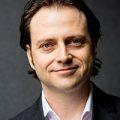
Each month I share a well-researched standpoint around CX, Insights and Analytics in my newsletter.
+4000 insights professionals read this bi-weekly for a reason.
I’d love you to join.





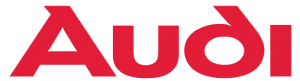
“It’s short, sweet, and practical.”
CX.AI -Experience the new generation of CX Insights
Rigor or Dogma?
About Theory-Led Research for Businesses … and Alternatives
Founder of CX-AI.com and CEO of Success Drivers
// Pioneering Causal AI for Insights since 2001 //
Author, Speaker, Father of two, a huge Metallica fan.
Author: Frank Buckler, Ph.D.
Published on: September 08, 2022 * 6 min read
Of cause, there is a reason why confirmatory research is so dominant in social sciences such as marketing science. Social matters are typically very complicated. When you see a correlation it does not mean there is a relationship or a causal link.
People tend to find reasons for correlations after seeing a correlation. But prior to the fact, the same person would have disregarded the hypothesis.
But when you have a hypothesis upfront that is based on a system of tested theories, and if then this hypothesis matches with the correlation measure after building the hypothesis, then – yes then the likelihood that the hypothesis is true is high.
The same approach still makes sense in multivariate models such as causal network models.
The example of the ancient philosopher HUME says: When a pool ball is struck by a stick – this could mean the stick causes the ball to move or the ball caused the stick to it them. Only your theory about pool billiards will tell you which version is more plausible.
Since now over 200 years this is the main idea behind social science. While being plausible, its practicability is so seldom questioned.
FREE for all client-side Insights professionals.
We ship your hardcopy to USA, CA, UK, GER, FR, IT, and ESP.
Yes, we all are hunting for the truth. A closer look will reveal that confirmatory approaches are well preferred in this circumstance:
If you have this at hand your confirmatory analysis will probably be the best method to use.
Sure if you are in doubt it might be wise to consider more explorative methods prior to using confirmatory methods. Here is a spectrum ranging from very explorative and qualitative to a quantitative approach that augments explorative with confirmatory approaches.
Most practitioners will agree: If you are new to a field, there is nothing better than talking face to face with customers in in-depth interviews. Yes, it is biased. But it helps you to understand holistically what might be important.
As always, the world is not black or white. There is something between pure qual and confirmatory quant.
There is a tendency to view confirmatory research as “better” than qualitative or quantitative explorative methods. Indeed, when the requirements are met, it is “better”.
Based on your experience, how often are confirmatory methods applied when actually requirements are clearly violated? Would you still use it if you would know a viable alternative?
David ran this customer satisfaction survey for SONOS. They reached out to every new customer one month after purchase. What David saw in the data was a big correlation between “excellent customer support” and loyalty/recommendation. It was validating what everybody believed. Not only that they build a multivariate model based on their hypotheses and well: the hypothesis was confirmed.
Taking a bit more explorative but still causal approach to the model (Causal Machine Learning) they included so-called context variables in the model. These are variables that may or may not explain outcomes or even moderate other effects.
Long story short: it turns out that customer support only correlates with (and “explains”) loyalty, because those buyers that already had SONOS speakers needed less support, and therefore, had less trouble, and naturally are more loyal and recommend more. The statistical ”effect” was spurious and no confirmatory approach could ever find this out. Why? Because it was missing the confounding variable “already existing customer” in the model.
Theory as well as “models are always wrong, some are useful” is a famous quote from George Cox.
Jordi was running the Marketing and Sales of Warsteiner – a leading German beer brand. They wanted to relaunch the beer case and needed to find out if sales would drop or even increase with a better case. Investing in a new beer case is a nine-figure investment.
A/B testing is a scientific confirmatory experiment and is seen as a highly valid method. Doing that they found that the new case would lose nearly 10% of sales.
A causal machine learning exercise however discovered something that (after the fact) made much more sense. The new case looks better and the customer like more in all relevant associations. But it lacks familiarity. The model showed that familiarity is one of the main drivers of purchase, which explains the drop in the A/B testing.
Now, any new design will lack familiarity. It grows over time, the more customers see the new design in the shops or in the commercials.
Now the cause-effect model could be used to understand this: When the new beer case design will be as familiar as the old beer case, the brand will sell 7% more, not 10% less.
A/B testing can be like comparing apples with oranges. If you sit on an imperfect hypothesis the rigor of your approach can be the cause of your failure.
Daniel was heading the commercial excellence program of SOLVAY – a pharmaceutical brand. He collected data on the activities of the salesforce and all marketing support activities. All this was fed into modeling to understand which actions drive most prescriptions.
One of the hypotheses of the confirmatory modeling was that providing product samples would drive prescriptions. But no matter how you tweak the modeling it always came to the conclusion: no significant impact.
Daniel tried a causal machine learning approach and was blown away by the elegance of the finding: Providing product samples has a nonlinear effect – an inverted U effect. Here is why.
If you provide samples it will help patients to try and eventually to use it long-term. However, if the physician has too many samples on stock, he becomes the sole source of the medication for more and more patience.
Some sales reps simply sampled too much, some not enough. Sampling makes sense but you need to have the right balance.
Nobody was hypothesizing the nonlinear effect. Still, causal machine learning could discover it and it turned out to be very useful.
There is a seldom shared pain in confirmatory modeling: often times coefficients turn out to be counterintuitive, e.g. showing a negative effect instead of a positive one.
Mel was running a CX program for insurance and she had this very same problem. “Excellent service” had a negative impact on the Likelihood-to-recommend. This made no sense at all.
Later it turned out that his confirmatory approach was the route cause. It is common practice to only include explaining variables in a model if there is a good hypothesis for its impact.
The point that this procedure totally misses is that eliminating a cause from a model is a hypothesis on its own. It assumes this cause has no impact.
Instead, it’s a good practice in causal machine learning to add “context information” to the model. For Mel it changed everything to add the customer segment as an explaining variable.
It turned out that there are segments with higher expectations which are having a lower likelihood to recommend at the same service level. While they have seen at times even better service levels, it leads “service” to correlate slightly negatively with the likelihood to recommend.
Due to including the segment, a Causal Machine Learning can derive from data, that the outcome offset is due to the segment but better service still improves the outcome.
It was 2013 when T-Mobile reinvented itself. The repositioning worked and the brand was growing. Only nobody exactly knew why. So they took a look at the brand tracking data. Modeling was done to reveal, which of the customer perceptions and service properties were causing the customer to come. With little success. No clear answer was available.
David – the head of insights at that time – was looking for fresh approaches and ran a causal machine learning exercise. The approach takes all information/variables available and structures it in a knowledge-led way: specific items about the service are seen as a potential cause. Purchase intent and consideration as an outcome. Other more vague items like brand perceptions are modeled as mediators. Also, context variables are included as potential moderators.
Someone had asked the team to include the item “T-Mobile is changing wireless for the better” into the tracker to measure whether or not the repositioning works.
As one of many items that could be a mediator, it was included as such in the model – not theory-led but “possibility-led”. This move changed the whole history of the company with a 6-fold market evaluation a few years later.
The analysis revealed that none of the changes like end-of-contract-binding, flat rate or free-iPhone were directly driving the customer to buy directly. It was that those actions perfectly reasoned why the new positioning is valid. This new positioning perception -to be the un-carrier- was what attracted customers. The learning was to continue introducing new features that reasoned the very same positioning.
Only a modeling approach that allows handling vague hypotheses in an explorative setting was able to discover what the company needed for its growth.
It seems that confirmatory research has some blind spots. You don’t know what you don’t know.
The question is if it would make sense to change the way we look at it: Instead of asking “what is the best approach in general”, why can’t we ask “what is the right approach right now?”
Confirmatory research brings most certainty and validity – only if the requirements are met.
More exploratory research is by design made to make us learn more, designed to discover new knowledge.
Sure, the discovery comes with failure, but as examples show us, confirmatory research too often provides illusory security.
Shouldn’t a researcher ask himself: Do I want to discover or do I really want to validate?
Write me!
p.s. Can “Causal AI” the new North star?
The recent Gartner Hypertrend Report now shows “CAUSAL AI” as one of the most promising technologies. It says that in 5 to 10 years the technology will be the tech that everyone needs to have to be not a laggard.
The two most promising platforms for Causal-AI are CausaLens which just received 50m funding and NEUSREL.com. While CausaLens is probably great for enjoying a good user interface, is -in my opinion- Neusrel technology-wise most advance.
At the end, you need to build your own opinion.
Trying out is the way to know better 😊
Each month I share a well-researched standpoint around CX, Insights and Analytics in my newsletter.
+4000 insights professionals read this bi-weekly for a reason.
I’d love you to join.
“It’s short, sweet, and practical.”