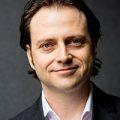
Each month I share a well-researched standpoint around CX, Insights and Analytics in my newsletter.
+4000 insights professionals read this bi-weekly for a reason.
I’d love you to join.





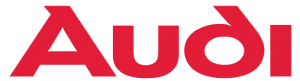
“It’s short, sweet, and practical.”
CX.AI -Experience the new generation of CX Insights
Founder of CX-AI.com and CEO of Success Drivers
// Pioneering Causal AI for Insights since 2001 //
Author, Speaker, Father of two, a huge Metallica fan.
Author: Frank Buckler, Ph.D.
Published on: September 11, 2023 * 7 min read
One speaks of synthetic market research when market research results are obtained purely from generative AI. But how can this be done? How is AI supposed to know what consumers are thinking here and now?
It is totally illogical. But what is useful is right. And so maybe it makes sense to take a closer look at the whole thing.
When I hear experts write about generative AI, people seem to divide into two camps. Those who are euphoric about the benefits of the new technology and then extrapolate this too euphorically into the future.
The other camp, while open to the use of technology (because the benefits within certain limits are obvious and no longer disputable), are downright happy to see any evidence of why technology is not as good as humans and existing techniques, and argue why this will remain so forever.
As always, the truth lies in the middle. Both the critical and the visionary mindset are needed. But according to Steve Jobs, only those who are “stupid” enough to think that this is impossible for a long time will change the world.
Logical, actually. If I assume that LLMs cannot replace market research, I will not be able to find out how and under which circumstances they might.
FREE for all client-side Insights professionals.
We ship your hardcopy to USA, CA, UK, GER, FR, IT, and ESP.
Earlier this year, a scientific paper by Harvard researchers made headlines. They used GPT3 and simulated the toothpaste purchasing process to find out what the price discount function looks like for different brands. Surprisingly, the results were quite close to conjoint measurement results
In February, another amazing study appeared in the Journal of Political Science in which GPT3 can be used to reproduce the results of political polls. I quote from the summary:
“We then compare the silicon and human samples to demonstrate that the information contained in GPT-3 goes far beyond surface similarity. It is nuanced, multifaceted, and reflects the complex interplay between ideas, attitudes, and sociocultural context that characterize human attitudes. We suggest that language models with sufficient algorithmic fidelity thus constitute a novel and powerful tool to advance understanding of humans and society across a variety of disciplines.”
In May, Steffen Schmidt from the Swiss LINK surprised with the use of an AgentGPT system that appreciated the price discounting function of the APPLE VISION PRO. The system takes a multi-step approach and first researches competitor prices, then promotes itself.
We at Success Drivers then tried to validate this in June by comparing the results with a “real” measurement of the price sales function using the Implicit Price Intelligence method (see Planning&Analysis issue 1/2023). Result: the resulting price-sales function differs, but the resulting optimal price is not that far from optimal. Simpler methods like Garbor Granger or Von-Westendorp would have been worse.
Kantar published a study on the use of synthetic market research in July and came to mixed conclusions. In essence, the researchers are trying to report the results of Likert scales. This works very well in some cases, not so well in others. In some cases, the researchers find strong demographic biases in the LLMs.
But there is also no shortage of critical articles.
Something to the effect of Gordon Guthrie‘s attempt to examine the reliability of the LLM by analyzing rare events. He and others see three fundamental weaknesses of the LLM (and leave their analysis at that)
This analysis is good and correct. But we will only find out if and how we can use the technology, if we consider it possible to use the technology -sensibly tamed or further developed-.
So I think about how the mind of a human being is knitted and I notice that it is quite similar to the mind of the LLM.
A nice example is this one. What does AI answer to these questions?:
The AI answers the way humans intuitively answer: “the student”. This answer is not politically correct nor logically unambiguous. But it is the probabilistically best answer based on the data we have learned.
The word “professor” is associated with “masculinity. We want to change that as a society, but that’s another story. The fact is that the human association is still also closer to reality.
The AI responds as a human would with its learned associations.
I conclude three theses from this:
THESIS 1 – Interviewing AI is similar to interviewing an individual human. It is not a database or structured knowledge. Think of the output of the AI as just ONE “opinions”.
THESIS 2 – We can simulate a representative survey if we ask (prompt) the AI to take the view of a specific human and run this for all variants of different consumers. These consumers can be described by his demographics, experiences, personality or values.
KASTEN: What’s interesting is that we have the chance to get more representative results than in real market research. Why? If I want to have 50% men and 50% East Germans in the sample, it can happen that in extreme cases all men come from East Germany. In other words: A necessary “multi-dimensional quotation” hardly ever takes place in market research practice for practical and cost reasons. But it is necessary in order to really ensure representativeness. In synthetic market research, this is simple, fast and free of charge. Of course, I need to know the actual distribution of the characteristics in reality, which will usually not be the case.
THESIS 3 – Validation is necessary: The following applies to synthetic market research (which should also apply to “real” horny): We cannot assume that market research results are correct unless we have validated them with other independent information.
Would you like an example?
Classic market research always produces results. But whether this can be taken at face value without reflection is another matter.
What are the validation methods? I can think of these three:
Why would a company take the risk of doing synthetic market research when you can buy a solid, real sample for a few hundred or thousand dollars?
A fair question: indeed, I suspect that synthetic market research is not a substitute for most of current market research practice.
But how often are there information needs in companies that are not covered by market research because it is too expensive and takes too long? Most decisions today are made on the basis of expert assessments, a few qualitative interviews and desktop research, and not on the basis of market research. Especially when you consider that about 50% of our economic value is created by companies with less than 50 million in sales.
Today, half of the economy hardly does professional market research. This raises the question of whether synthetic market research can complement and improve expert opinions, “self-service SurveyMonkey”, a few qualitative interviews or desktop research.
There are also several phases in the insight process of large companies. It starts with a qualitative phase and the quantitative phase, in which investment is made in market research, only begins once the options space has been narrowed down.
Especially in the preliminary phase, it can make a lot of sense to use synthetic market research. Because it can complement the methods used and improve their output.
As my doctoral advisor Klaus-Peter Wiedmann used to say? “Mr. Buckler, do one thing, don’t do another”.
We humans still have a distorted picture of what LLMs actually are. They are not “artificial humans” and not databases and neither work accurately nor are they always right. They are, like the human mind, nothing else than an association machine, which -like us- has learned with non-representative data and which -like us- talks smart, but what the whole value is, a validation has to show.
With this picture in mind, it may be possible to use LLMs for tasks that are bungled today.
Today, the market research industry has the opportunity to occupy the topic for itself and thus create new markets. If it doesn’t do it, others will.
Let’s get it done. Or as Klaus-Peter used to say “there is nothing good unless you do it”.
Her,
Frank Buckler
Author:
Dr. Frank Buckler is founder and managing director of Success Drivers GmbH a marketing research agency focusing on the application of AI in gaining insights for marketing and sales. He has been researching AI for 30 years and is the developer of the AI-based causal analysis software NEUSREL. Since the publication of the book “Neuronale Netzte im Marketing-Management” in 2001 by Gabler/Springer, he is a frequent book author in the field of AI and marketing.
p.s. I have left out of this article that the human being and even the human brain as a whole differs from the LLM in many other aspects. But we will discuss that in another issue of the Future Technologies column.
LITERATURE
Each month I share a well-researched standpoint around CX, Insights and Analytics in my newsletter.
+4000 insights professionals read this bi-weekly for a reason.
I’d love you to join.
“It’s short, sweet, and practical.”